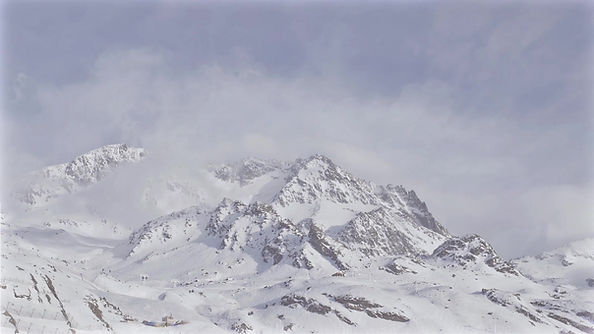
What are you doing with your data today?
Explore Our Services: Discover the Solutions We Offer! Interested in learning more? Connect with us today for further details and personalized assistance
Services
Dathabor provides several services that can be obtained by contracting work (1-5 days a week) or project-based work. From the roles mentioned below in the services section, a mixture of services can also be provided. This will mostly be depending on your company size and current data organization. Feel free to contact us for more details, or to discuss your specific requirements.
Data Analyst
For the role of Data Analyst, Dathabor provides the following set of activities. Dathabor can help you in any of those activities, as a fixed contractor, or for specific project requirements.
​
-
Data Exploration: Exploring and understanding datasets to identify patterns, trends, and relationships that can provide valuable insights into business performance and operations.
-
Data Cleaning and Preprocessing: Cleaning and preprocessing raw data to ensure accuracy, consistency, and completeness, which involves handling missing values, outliers, and inconsistencies in the data.
-
Descriptive Analysis: Conducting descriptive analysis to summarize and visualize key metrics and KPIs, such as sales trends, customer behavior, or website traffic, using techniques like data visualization and summary statistics.
-
Diagnostic Analysis: Investigating the underlying factors driving trends and patterns observed in the data, such as identifying root causes of performance issues or anomalies, using techniques like root cause analysis and hypothesis testing.
-
Predictive Modeling: Building predictive models to forecast future outcomes or trends based on historical data, using techniques such as regression analysis, time series forecasting, or machine learning algorithms.
-
Prescriptive Analysis: Recommending actions or interventions based on insights derived from data analysis, such as identifying opportunities for cost reduction, revenue optimization, or process improvement.
-
Statistical Analysis: Applying statistical techniques and methods to analyze data and test hypotheses, such as hypothesis testing, correlation analysis, or A/B testing, to draw meaningful conclusions and make data-driven decisions.
-
Data Interpretation and Reporting: Interpreting analysis results and preparing reports or presentations to convey findings to non-technical stakeholders, such as business leaders or clients, in a clear and understandable manner.
-
Collaboration and Communication: Collaborating with cross-functional teams, including business stakeholders, data engineers, and data scientists, to understand business requirements and ensure alignment between data analysis efforts and business objectives. Communicating findings, insights, and recommendations to stakeholders through written reports, presentations, or verbal discussions.
-
Continuous Learning and Improvement: Staying updated on the latest tools, techniques, and trends in data analysis and related fields. Seeking opportunities for continuous learning and skill development to enhance analytical capabilities and deliver greater value to the organization.
Data Engineer
For the role of Data Engineer, Dathabor provides the following set of activities. Dathabor can help you in any of those activities, as a fixed contractor, or for specific project requirements.
​
-
Data Pipeline Development: Designing, building, and maintaining robust data pipelines to efficiently move data from various sources to destination systems, ensuring reliability, scalability, and performance.
-
Data Integration: Integrating data from disparate sources such as databases, APIs, files, and streaming platforms, and transforming it into a unified format for storage and analysis.
-
Database Management: Setting up and managing databases and data warehouses, including installation, configuration, tuning, and optimization of database systems such as SQL, NoSQL, or NewSQL databases.
-
ETL (Extract, Transform, Load) Processes: Developing and maintaining ETL processes to extract data from source systems, transform it according to business requirements, and load it into target systems for storage, analysis, and reporting.
-
Big Data Technologies: Working with big data technologies such as Hadoop, Spark, and Hive to process and analyze large volumes of data efficiently, leveraging distributed computing and storage capabilities.
-
Cloud Data Platforms: Utilizing cloud-based data platforms such as AWS, Azure, or Google Cloud to build and deploy data solutions, taking advantage of managed services for storage, compute, analytics, and machine learning.
-
Data Quality Assurance: Implementing data quality checks, validation rules, and monitoring processes to ensure data accuracy, completeness, consistency, and integrity throughout the data lifecycle.
-
Performance Optimization: Optimizing the performance of data systems and processes by tuning queries, indexing strategies, partitioning schemes, and resource allocation to improve efficiency and reduce latency.
-
Data Security and Compliance: Implementing security measures such as encryption, access controls, and audit trails to protect sensitive data, as well as ensuring compliance with data privacy regulations and industry standards.
-
Collaboration and Documentation: Collaborating with cross-functional teams including data scientists, analysts, and business stakeholders to understand requirements and deliver solutions that meet business needs. Documenting data processes, workflows, and configurations to ensure knowledge sharing and maintain system documentation.
Data (BI) Consultant
For the role of Data (BI) Consultant, Dathabor provides the following set of activities. Dathabor can help you in any of those activities, as a fixed contractor, or for specific project requirements.
​
-
Business Needs Assessment: Conducting comprehensive assessments of clients' business needs, objectives, and challenges to understand their requirements and goals for BI and analytics solutions.
-
BI Strategy Development: Developing tailored BI strategies and roadmaps aligned with clients' business objectives, outlining the vision, goals, and steps required to leverage data for informed decision-making and business optimization.
-
Data Analysis and Visualization: Performing data analysis to extract meaningful insights and trends from complex datasets, and creating interactive visualizations, dashboards, and reports using tools such as Tableau, Power BI, or QlikView.
-
Data Modeling and Architecture: Designing and implementing data models and architectures that support efficient storage, retrieval, and analysis of data, ensuring scalability, performance, and usability for BI and analytics applications.
-
Performance Optimization: Optimizing the performance of BI systems, queries, and reports through query tuning, indexing strategies, and optimization of data models and architectures to enhance responsiveness and usability.
-
User Training and Adoption: Providing training and support to end-users to enhance their proficiency in using BI tools and applications, and driving user adoption of BI solutions to maximize their value and impact on business operations.
-
Continuous Improvement and Optimization: Monitoring and evaluating the effectiveness of BI solutions, and identifying opportunities for continuous improvement and optimization to meet evolving business needs and technology advancements.
-
Stakeholder Collaboration and Communication: Collaborating with stakeholders across the organization, including business leaders, analysts, and IT teams, to ensure alignment of BI initiatives with business goals and priorities. Communicating findings, insights, and recommendations to stakeholders through presentations, reports, and workshops.
-
Vendor Selection and Implementation: Assisting clients in selecting appropriate BI tools and technologies based on their requirements and budget, and managing the implementation and deployment of BI solutions to ensure successful adoption and integration with existing systems and processes.
​
​
Data Architect
For the role of Data Architect, Dathabor provides the following set of activities. Dathabor can help you in any of those activities, as a fixed contractor, or for specific project requirements.
​
-
Requirement Analysis: Collaborating with stakeholders to understand the organization's business objectives, data requirements, and technical constraints to inform the design of the data architecture.
-
Data Modeling: Designing conceptual, logical, and physical data models that define the structure, relationships, and attributes of the organization's data assets, ensuring alignment with business objectives and data governance standards.
-
Database Design and Management: Selecting and designing appropriate database technologies and systems (e.g., relational databases, NoSQL databases, data warehouses) based on data requirements, scalability needs, and performance considerations. Managing database schemas, configurations, and optimizations to ensure efficient data storage, retrieval, and management.
-
Data Integration and ETL: Designing data integration solutions and ETL (Extract, Transform, Load) processes to consolidate, cleanse, and transform data from disparate sources into a unified format for analysis and reporting. Implementing data integration pipelines and workflows to automate data movement and synchronization.
-
Data Governance and Compliance: Establishing data governance policies, standards, and procedures to ensure data quality, integrity, security, and compliance with regulatory requirements (e.g., GDPR, HIPAA). Implementing data access controls, encryption, and auditing mechanisms to protect sensitive data assets.
-
Big Data and Cloud Architecture: Designing scalable and resilient data architectures for big data and cloud environments, leveraging technologies such as Hadoop, Spark, Kafka, and cloud-based services (e.g., AWS, Azure, Google Cloud) to process, store, and analyze large volumes of data.
-
Metadata Management: Implementing metadata management solutions to capture and maintain metadata (e.g., data definitions, lineage, usage) for data assets, providing transparency and traceability for data stakeholders and ensuring data lineage and impact analysis capabilities.
-
Data Security and Privacy: Assessing and mitigating data security risks by implementing encryption, access controls, and data masking techniques to protect sensitive data from unauthorized access or disclosure. Ensuring compliance with data privacy regulations and industry standards.
-
Data Architecture Documentation: Documenting the data architecture design, components, standards, and best practices to facilitate knowledge sharing, collaboration, and consistency across data-related initiatives and projects.
-
Collaboration and Stakeholder Engagement: Collaborating with cross-functional teams, including business stakeholders, data engineers, data scientists, and IT professionals, to align data architecture initiatives with business goals and requirements. Engaging with stakeholders to gather feedback, prioritize requirements, and communicate architectural decisions.
-
Technology Evaluation and Road mapping: Evaluating emerging technologies, tools, and platforms relevant to data architecture, and recommending adoption strategies and roadmaps based on business objectives, technical feasibility, and industry trends.
Data Scientist
For the role of Data Scientist, Dathabor provides the following set of activities. Dathabor can help you in any of those activities, as a fixed contractor, or for specific project requirements.
​
-
Problem Framing: Collaborating with stakeholders to define business problems and objectives that can be addressed through data analysis and machine learning, ensuring alignment with strategic goals and priorities.
-
Data Collection and Preparation: Gathering, extracting, and preprocessing data from various sources (e.g., databases, APIs, files) to create clean, structured datasets suitable for analysis and modeling.
-
Exploratory Data Analysis (EDA): Exploring and visualizing data to understand patterns, trends, and relationships, using statistical methods and visualization tools to gain insights into the underlying data distribution and characteristics.
-
Feature Engineering: Creating new features or transforming existing features to enhance the predictive power of machine learning models, leveraging domain knowledge and statistical techniques to engineer relevant features from raw data.
-
Model Selection and Training: Selecting appropriate machine learning algorithms and techniques based on the nature of the problem and data characteristics, training and evaluating models using techniques such as cross-validation and hyperparameter tuning to optimize performance.
-
Predictive Modeling: Building predictive models to forecast future outcomes, classify data into categories, or detect anomalies, using techniques such as regression, classification, clustering, and time series analysis.
-
Model Interpretation and Evaluation: Interpreting model results and evaluating model performance using metrics such as accuracy, precision, recall, F1-score, and ROC-AUC, and validating model assumptions to ensure robustness and reliability.
-
Deployment and Integration: Deploying trained models into production environments and integrating them into business processes or applications, ensuring scalability, reliability, and maintainability of deployed solutions.
-
Model Monitoring and Maintenance: Monitoring model performance and behavior in production, detecting drifts or degradation in model performance over time, and retraining or updating models as needed to maintain their effectiveness.
-
Experimentation and A/B Testing: Designing and conducting experiments or A/B tests to evaluate the impact of data-driven interventions or model-driven decisions on business outcomes, using statistical methods to analyze and interpret experimental results.
-
Collaboration and Communication: Collaborating with cross-functional teams, including business stakeholders, data engineers, and software developers, to translate data science insights into actionable recommendations and communicate findings effectively through reports, presentations, or dashboards.
-
Continuous Learning and Skill Development: Staying updated on the latest developments in data science, machine learning, and related fields, acquiring new skills and knowledge through self-study, online courses, or participation in conferences and workshops.
Contact
Like what you see? Get in touch to learn more.